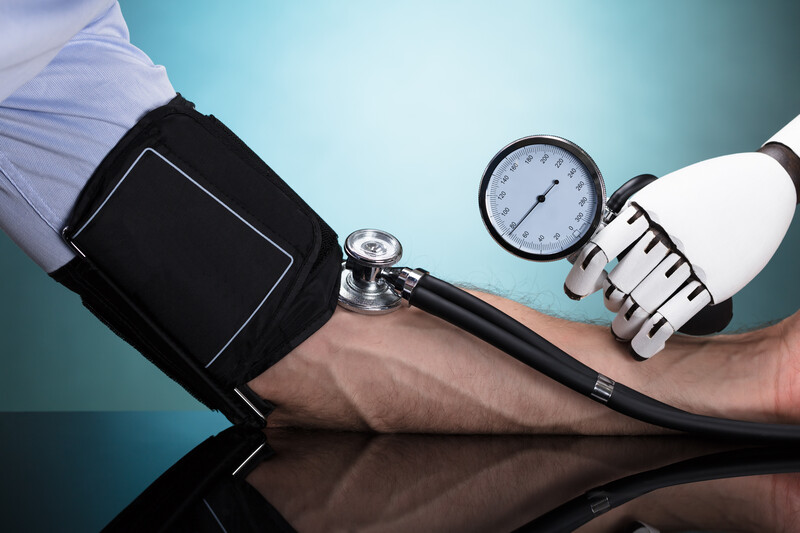
FDA Action Plan for AI/ML in SaMD (Software as a Medical Device)
Artificial intelligence (AI) and machine learning (ML) technologies have a huge potential to change the way we look at our current health care practices. AI/ML enable new insights derived from the huge amount of data that is collected every day in the health care sector. This blog provides an overview of the FDA action plan for AI/ML in software as a medical device and lists some best practices.
Software algorithms can be used to learn from real-world applications to improve a product’s performance. However, they present a set of new considerations due to the complexity in their development and the data that is collected.
In January 2021 the FDA released an action plan titled Artificial Intelligence/Machine Learning (AI/ML)-Based.
This plan was developed in direct response to feedback from stakeholders to provide practical oversight and future considerations the FDA will make for AI/ML based Medical Devices. The FDA’s traditional paradigm of medical device regulation was not designed for adaptive artificial intelligence and machine learning technologies. Under their current approach to software modifications, the FDA anticipates that many of these AI/ML changes to a device may need a premarket review.
As part of the action plan, the FDA highlights 6 key considerations manufacturers should make when developing AI/ML based SaMD.
1) Principle of predetermined change control plan – the FDA would like to know what aspects the manufacturer intends to change through learning, and the algorithm change protocol – how the algorithm will learn and change while remaining safe and effective.
2) Proactive patient-centered approach to development and utilization that takes into account issues including usability, equity, trust, and accountability. One way that the FDA is addressing these issues is through the promotion of transparency of these devices to users and patients that mostly pertains to the devices’ functionality
3) Labeling for AI/ML-based devices and the need for manufacturers to clearly describe the data that used to train the algorithm, the relevance of its inputs, the logic it uses (if possible), the role intended to be served by its output, and the evidence of the device’s performance.
4) Account for bias and generalizability. This is not an issue exclusive to AI/ML-based devices. Because AI/ML systems are developed and trained using data from historical datasets, they are vulnerable to bias – and AI/ML is prone to mirroring biases present in the data. Healthcare delivery is known to vary by factors such as race, ethnicity, and socio-economic status; therefore, it is possible that biases currently present in a health care system may be inadvertently introduced into the algorithms.
The FDA believes it’s important for medical devices to be well suited for a racially and ethnically diverse intended patient population. This includes the need for improved methodologies for identification and improvement of ML algorithms.
5) The FDA would like manufacturers to consider gathering Real World Performance (RWP). Gathering performance data on the real-world use of the SaMD can allow manufacturers to understand how their products are being used, identify opportunities for improvements, and respond proactively to safety or usability concerns. Real-world data collection and monitoring can be used by manufacturers to leverage mitigating the risk involved with AI/ML-based Software modifications.
6) In October 2021, the FDA together with Health Canada and the UK’s Medicines and Healthcare products Regulatory Agency (MHRA) jointly identified 10 guiding principles that can inform the development of Good Machine Learning Practices or GMLP to describe a set of AI/ML best practices. These 10 principles are as follows;
- Multi-Disciplinary Expertise Leveraged Throughout the Total Product Life Cycle
- Good Software Engineering and Security Practices Are Implemented
- Clinical Study Participants and Data Sets Are Representative of the Intended Patient Population
- Training Data Sets Are Independent of Test Sets
- Selected Reference Datasets Are Based Upon Best Available Methods
- Model Design Is Tailored to the Available Data and Reflects the Intended Use of the Device
- Focus Is Placed on the Performance of the Human-AI Team
- Testing Demonstrates Device Performance during Clinically Relevant Conditions
- Users Are Provided Clear, Essential Information
- Deployed Models Are Monitored for Performance and Re-training Risks Are Managed
More info on this list can be found in https://www.fda.gov/media/153486/download
By following the FDA’s updates on the AI/ML-based SaMD action plan, medical device manufacturers planning to market in the US can position themselves to understand and embrace the new regulations and avoid mistakes or a last-minute rush to achieve compliance. The FDA provides a list of AI/ML enabled medical devices marketed in the United States as a resource to the public. This list can be found here: Artificial Intelligence and Machine Learning (AI/ML)-Enabled Medical Devices.
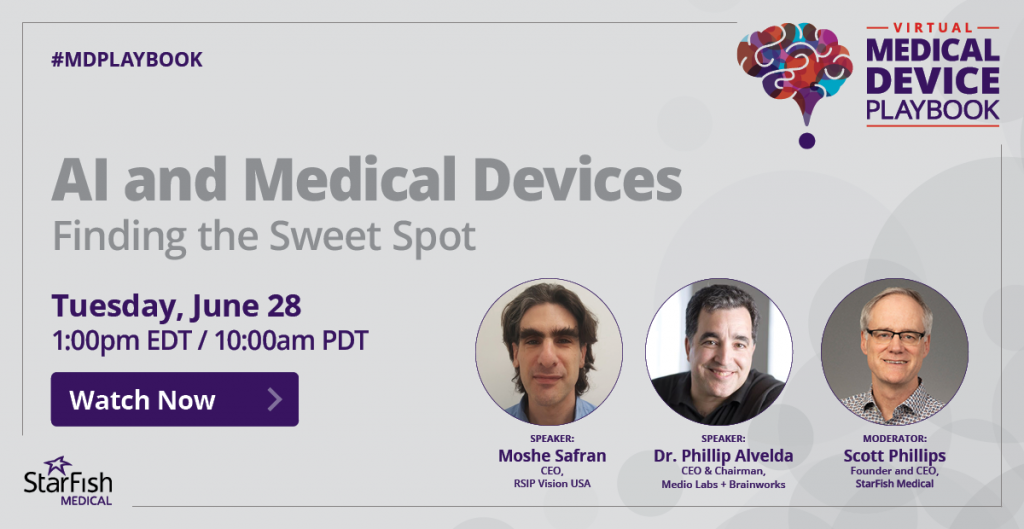
(c) Can Stock Photo / AndreyPopov
Taimoor Khan is a QA/RA Specialist at StarFish Medical. Based in our Toronto office, Taimoor works on a variety of medical device design & product development https://starfishmedical.com/services/product-design-development/projects. He follows his own advice on ROHS and REACH management and qualification regularly.